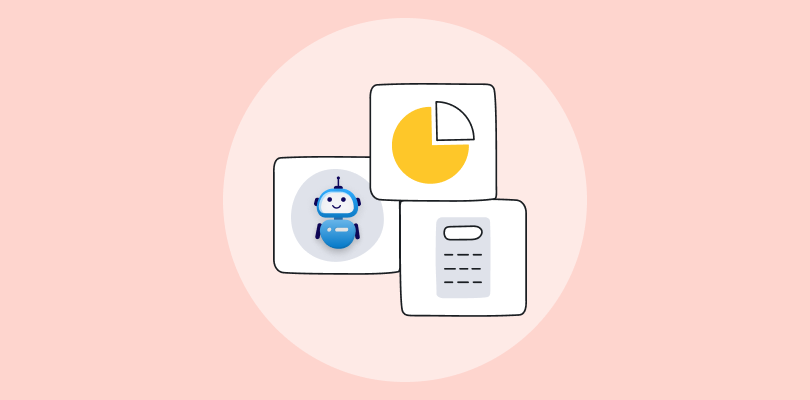
Chatbots have gained a lot of popularity, transforming how businesses interact with customers.
But how do you know if your chatbot is a hero, saving the day with efficient service and happy interactions, or a silent villain, frustrating users and failing to meet expectations?
The answer lies in data.
By tracking key chatbot analytics metrics, you can gain valuable insights into bot performance and user behavior and identify areas for improvement.
In this blog, I’ll unveil the top 20 metrics for chatbot analytics you can track to optimize your chatbot for success. But before we get a detailed view of each metric, here is a sneak peek of each with their meaning.
Metric | What it Indicates |
---|---|
Total Chats | Number of chats per session |
Conversion Rate | Percentage of users taking the desired action |
Session Duration | The average length of a session |
User Retention Rate | Percentage of returning users |
Fall-Back Rate | Frequency of default or escalated responses |
Goal Completion Rate (GCR) | Percentage of successfully completed tasks |
Response Accuracy | Accuracy of responses given |
User Satisfaction Score | Average user satisfaction rating |
Active Users | Number of unique users in a time frame |
Bounce Rate | Percentage of one-time interactions |
Intent Recognition Rate | Accuracy of intent recognition |
Chatbot Efficiency | Average response time per query |
Cost Per Conversation | Average cost per conversation |
Escalation Rate | Percentage of conversations escalated to humans |
Message Open Rate | Percentage of messages opened |
Churn Rate | Percentage of users who discontinue use |
Net Promoter Score (NPS) | Level of user loyalty and recommendation likelihood |
Multilingual Effectiveness | Effectiveness across different languages |
Query Resolution Time | Average time to resolve a query |
Feedback Rate | Frequency of feedback provided |
Popular Chatbot Analytics to Track for Delightful Interactions
1. Total Chats
Total Chat is a metric that counts the total number of conversations between users and a chatbot. This indicator is crucial for analyzing a chatbot’s performance as it directly reflects its engagement level and accessibility.
Higher chat volumes often suggest better visibility and utility, making them a foundational metric for evaluating user interest and the effectiveness of the chatbot’s deployment.
Studies show that a chatbot that successfully engages users can increase customer satisfaction by 20%.
Also Read: How to Boost Customer Engagement Using Live Chat
2. Conversion Rate
The conversion rate tracks the percentage of chat sessions that result in the user completing a predefined goal, such as purchasing, registering for an event, or subscribing to a service. This metric is vital for evaluating the effectiveness of the chatbot in driving business outcomes.
For instance, a chatbot that collects sign-ups for a webinar could measure how many conversations lead to registrations.
Chatbots have been found to improve conversion rates by up to 40% when effectively implemented.
3. Session Duration
Session duration measures the average time a user interacts with the chatbot during a single session. This metric provides insights into how engaging and efficient the chatbot is. If users spend a long time interacting with the bot, it could indicate either strong engagement or possible confusion and inefficiency in its design.
For example, analyzing how long users interact with a customer service bot before their query is resolved can provide valuable insights.
Lengthy sessions may signal complicated or problematic interactions, necessitating further refinement.
4. User Retention Rate
User retention rate is the percentage of users who return to interact with the chatbot over a designated period. This metric is crucial for assessing the chatbot’s long-term value and effectiveness in maintaining user interest.
For example, measuring the number of users who repeatedly consult a health advisory chatbot over a month can indicate its usefulness and reliability.
A high retention rate often signifies a successful chatbot, sometimes maintaining over 50% return user rate.
Also Read: 15 Customer Retention Strategies That Actually Work
5. Fall-Back Rate
The fall-back rate calculates how frequently the chatbot cannot understand user queries and resorts to default answers or escalation to human assistance. This metric is important for measuring the chatbot’s ability to handle inquiries effectively.
For instance, tracking how often a travel booking bot defaults to human assistance when asked to book flights can highlight areas for improvement.
Keeping the fall-back rate below 10% indicates a capable and efficient chatbot.
6. Goal Completion Rate (GCR)
Goal Completion Rate measures how well the chatbot achieves the specific objectives it was designed for, such as assisting with account management or facilitating transactions. High GCR indicates a functional and effective bot.
For example, a bot tasked with helping users reset their passwords would closely monitor the number of password resets that are successfully executed.
Enhancing GCR by just a few percentage points can lead to a significant increase in user satisfaction.
Also Read: 14 Live Chat Metrics & KPIs to Measure Operator Performance
7. Response Accuracy
Response accuracy is a feature of a chatbot that assesses whether the chatbot’s answers are correct and relevant to the user’s queries. This metric is crucial for maintaining user trust and ensuring a high-quality interaction.
For example, evaluating whether a weather bot consistently provides accurate weather forecasts when queried is essential for user reliance on the service.
A response accuracy rate above 80% is crucial for maintaining user trust and satisfaction.
8. User Satisfaction Score
The user satisfaction score is often obtained through post-interaction surveys where users rate their experience on a scale. This feedback is integral for assessing how well the chatbot meets user needs and expectations.
For instance, a chatbot used for technical support might ask users to rate their satisfaction after a support session.
Approximately 70% of users report higher satisfaction when chatbots resolve their issues efficiently.
9. Active Users
This metric counts the number of unique users interacting with the chatbot within a specific timeframe, such as daily, weekly, or monthly. Tracking active users helps gauge the chatbot’s reach and overall user interest.
For example, monitoring how many people engage with a fitness coach bot daily can provide insights into its effectiveness and user engagement levels.
Successful chatbots can experience rapid growth in active users, sometimes seeing increases of 50% month-over-month after launch.
10. Bounce Rate
Bounce rate is the percentage of users who leave the chat after only a single interaction. This metric is crucial for understanding the impact and effectiveness of the chatbot’s greeting or first response. High bounce rates might suggest that users do not find the bot engaging or relevant to their needs.
For instance, calculating how many users leave after the first interaction with a bot designed for event information can indicate the clarity and appeal of the bot’s introductory message.
A bounce rate lower than 40% is typically good, indicating that the bot successfully engages most new users.
11. Intent Recognition Rate
The intent recognition rate measures the chatbot’s ability to accurately understand and categorize the user’s intent based on input. This is critical for providing relevant and accurate responses.
For example, assessing how well a customer support bot recognizes and differentiates between product return requests and product support can significantly impact service quality.
Advances in natural language processing have enabled chatbots to achieve intent recognition accuracies of over 85% in robust systems.
12. Chatbot Efficiency
Chatbot efficiency measures the promptness and speed with which a chatbot responds to user queries. This metric is important for user satisfaction as it reflects the chatbot’s capability to provide quick solutions. Timing how long it takes for a bot to provide a correct answer to a simple customer query can reveal a lot about its efficiency.
Efficient chatbots have been shown to reduce response times by up to 70%, significantly improving user experience.
Read
Read: Top 10 Free AI Chatbot Software in 2024 [With Pros & Cons]
13. Cost Per Conversation
Cost per conversation analyzes the chatbot’s economic impact by dividing the total operational costs by the number of conversations handled. This metric helps understand the chatbot’s cost.
For example, analyzing the operational costs of a customer service bot in relation to the number of inquiries it processes can help determine its financial efficiency.
Implementing chatbots can reduce the costs of customer service operations by up to 30%, proving them a cost-effective alternative to traditional methods.
14. Escalation Rate
The escalation rate tracks how often interactions with the chatbot are escalated to human operators. This is a critical metric for understanding the chatbot’s capability to handle complex queries independently.
For instance, measuring how often a technical support bot escalates issues to human technicians can provide insights into its effectiveness and limitations.
Keeping the escalation rate under 10% is generally considered ideal, ensuring the bot handles most queries efficiently.
Also Read: First Contact Resolution: How to Measure and Improve It
15. Message Open Rate
For chatbots that send proactive notifications or messages, the message open rate measures how often users actually open these communications. This metric indicates the relevance and timing of the messages sent. Tracking the open rates of promotional messages a retail bot sends during a sale event can help optimize future marketing strategies.
Open rates for messages sent by chatbots can reach 80-90%, significantly outperforming email open rates.
16. Churn Rate
The churn rate quantifies the percentage of users who stop interacting with the chatbot after an initial period of use. This metric is vital for assessing the chatbot’s long-term appeal.
For example, tracking the number of users who discontinue using a budgeting bot after a few sessions can help identify potential areas for improvement in user engagement or functionality.
A high churn rate can signal significant issues with the chatbot’s user experience or value proposition, necessitating a thorough review and adjustment.
Also Read: 9 Best Customer Retention Software Tools to Reduce Churn Rate
17. Net Promoter Score (NPS)
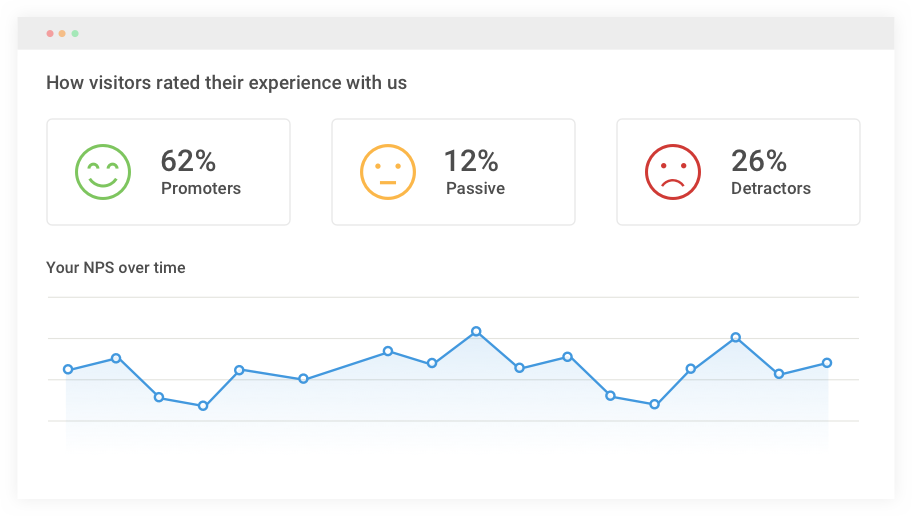
Net Promoter Score is a widely used metric that measures customer loyalty and satisfaction based on how likely users are to recommend the chatbot to others. An NPS survey typically asks users to rate their likelihood of recommending the service on a scale from 0 to 10. High scores indicate strong user loyalty and satisfaction.
For instance, a chatbot could ask users about their recommendation likelihood at the end of a service interaction.
An NPS score above 50 is excellent and indicates that users are highly likely to recommend the chatbot.
Also Read: 7 Customer Satisfaction Metrics You Should Measure
18. Multilingual Effectiveness
This metric evaluates how effectively the chatbot handles interactions in different languages, which is crucial for global applications. It assesses both the accuracy of language processing and the cultural appropriateness of responses.
For example, measuring user satisfaction and response accuracy in different language settings of a customer service bot can highlight its global usability and effectiveness.
Providing support in multiple languages can boost user engagement significantly in regions with diverse linguistic backgrounds.
19. Query Resolution Time
Query resolution time measures the average duration it takes for the chatbot to resolve a user’s query. This metric is essential for assessing the chatbot’s efficiency in providing timely solutions.
For instance, tracking the time from when a user asks a question to when they receive a satisfactory answer can indicate the bot’s performance.
Ensuring a quick resolution time, especially on mobile devices, is crucial for maintaining high user satisfaction with chatbots.
Related: What is an AI Chatbot
20. Feedback Rate
The feedback rate measures how often users provide feedback on their chatbot experience through ratings, comments, or direct feedback tools. This metric is key for continuous improvement and user-centric optimization of the chatbot.
For example, a flight booking chatbot could track the percentage of users who provide feedback after completing a booking, offering insights into user satisfaction and areas for improvement.
Only about 10% of users normally leave feedback, but even this small proportion can provide valuable insights for enhancing the chatbot’s performance.
Also Read: Chat Rating: Best Way to Get Customer Feedback
Why Is ProProfs Chat the Top Choice for Analyzing Chatbot Analytics?
ProProfs Chat is highly efficient in presenting visually rich bot performance analytics that are easy to comprehend. It offers a real-time monitoring dashboard, which allows businesses to observe chatbot interactions as they happen, providing immediate insights into visitor engagement and bot efficiency.
Besides, the platform includes detailed reporting tools that generate actionable data on chatbot performance, including response accuracy and user satisfaction. It also supports integration with other analytics tools, enhancing its capability to deliver deeper data analysis. These features make it a top choice for businesses looking to optimize their chatbot strategies and improve customer service efficiency.
Learn More About Chatbot Analytics
How can businesses use chatbot analytics to improve customer interactions?
Businesses can use chatbot analytics to enhance customer interactions by analyzing data on user engagement and feedback. This allows for identifying common issues or questions, enabling companies to adjust their chatbot’s responses for clarity and effectiveness. Additionally, analyzing user paths through conversation flows can help optimize the journey to reduce drop-offs and improve conversion rates, thereby enhancing the overall customer experience.
What role does artificial intelligence play in chatbot analytics?
Artificial intelligence (AI) plays a crucial role in chatbot analytics by enabling the automated parsing and understanding of large volumes of chat data. AI technologies, such as natural language processing, help identify patterns, sentiment, and context within conversations, which assists in refining the chatbot’s accuracy and responsiveness. AI can also predict user behavior and personalize interactions, making chatbots more effective in handling diverse user needs.
How frequently should businesses analyze chatbot data to drive insights and improvements?
The frequency of analyzing chatbot data should align with business goals and the volume of interactions. For dynamic sectors or during initial deployment, weekly reviews may be necessary to quickly adapt and optimize chatbot functionalities. Monthly or quarterly analyses might suffice for more established systems, allowing businesses to gather substantial data for meaningful insights and to plan strategic enhancements based on trends and user feedback.
FREE. All Features. FOREVER!
Try our Forever FREE account with all premium features!